In an article recently submitted to the Arxiv* preprint server, researchers at Google Research, the Weizmann Institute, Tel-Aviv University, and Technion introduced Lumiere, a text-to-video (T2V) diffusion model designed for realistic and coherent motion synthesis in videos. Utilizing a space-time U-Net architecture, Lumiere generated the entire temporal duration of a video in a single pass, distinguishing itself from existing models by achieving global temporal consistency through spatial and temporal down- and up-sampling.
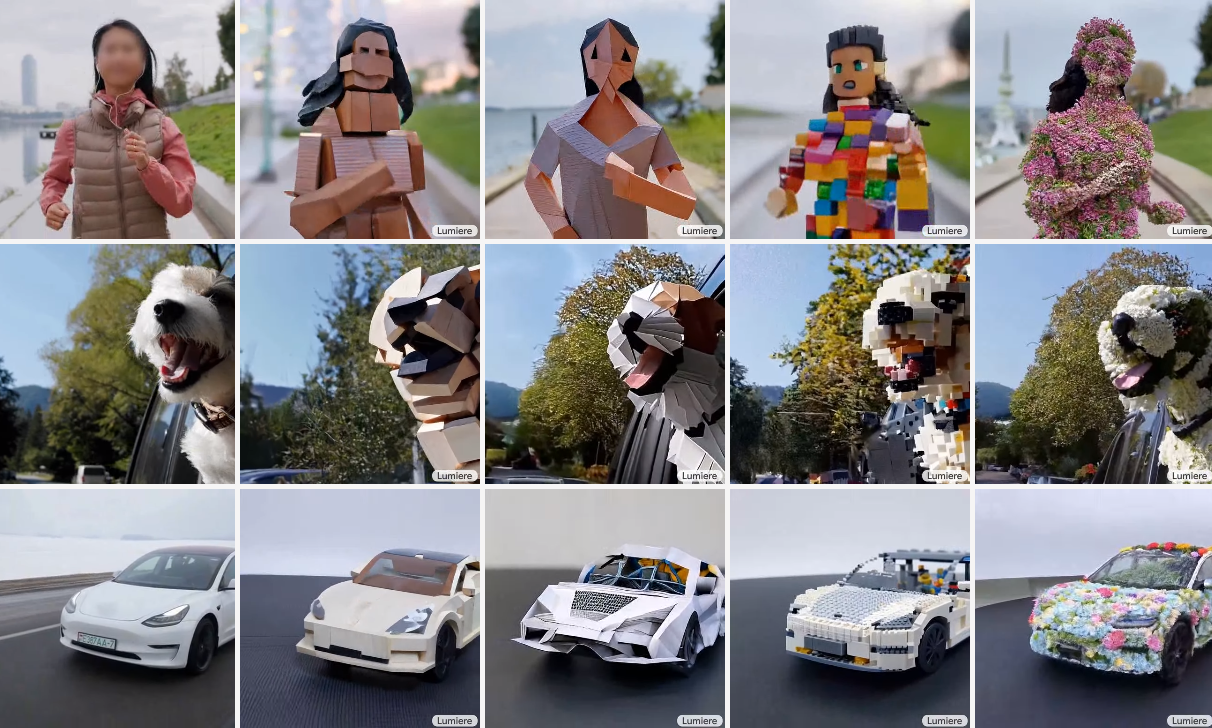
Study: Lumiere: A Space-Time Diffusion Model for Video Generation

*Important notice: arXiv publishes preliminary scientific reports that are not peer-reviewed and, therefore, should not be regarded as definitive, used to guide development decisions, or treated as established information in the field of artificial intelligence research.
Background
Generative models for text-to-image (T2I) diffusion have made significant strides, enabling the synthesis of high-resolution, realistic images from complex text prompts. However, extending this capability to T2V models presents challenges due to the complexities introduced by motion. Existing T2V models often adopt cascaded designs, generating distant keyframes and using temporal super-resolution (TSR) models for interpolation. This approach, while memory-efficient, struggles with global temporal coherence.
The proposed paper introduced a novel T2V diffusion framework using a space-time U-Net architecture to generate the full temporal duration of a video at once. Unlike conventional T2V models, the new approach incorporated both spatial and temporal down-sampling, optimizing computation in a compact space-time representation. Leveraging a pre-trained T2I model, Lumiere, the proposed T2V model addressed limitations in video duration, overall visual quality, and realistic motion generation.
By introducing global continuity through Multidiffusion, the researchers demonstrated state-of-the-art video generation, showcasing adaptability for various content creation tasks such as video inpainting, image-to-video generation, and stylized video production. The innovative design allowed for consistent editing using off-the-shelf methods, offering a promising solution to the challenges faced by existing T2V models.
The Lumiere T2V Model
The authors introduced Lumiere, a T2V diffusion model utilizing diffusion probabilistic models trained to approximate data distribution through denoising steps. Lumiere incorporated a base model and a spatial super-resolution (SSR) model, where the base model generated video clips at a coarse spatial resolution, and the SSR model spatially up-sampled the output, yielding a high-resolution video.
To address computational challenges, the authors proposed a space-time U-Net architecture, which performed most computations in a compact space-time representation by interleaving temporal blocks. Training the added parameters while keeping the T2I weights fixed, the model maintained equivalence to the pre-trained T2I model at initialization. The Multidiffusion technique was employed to smoothen transitions between temporal segments, ensuring coherence in SSR predictions over overlapping windows.
Lumiere demonstrated state-of-the-art video generation, offering a versatile framework applicable to various content creation tasks, including video inpainting, image-to-video generation, and stylized video production. The proposed design enhanced computational efficiency and preserved global temporal consistency, addressing limitations in existing T2V models.
Applications
The absence of temporal cascades enhanced global temporal consistency, making Lumiere suitable for various downstream applications. Lumiere's application scope included stylized generation, where a "plug-and-play" approach combined T2I weights and style interpolation for realistic motion across diverse styles. The model excelled in conditional generation, accommodating additional input signals such as images or masks, enabling image-to-video synthesis, inpainting for object replacement or localized editing, and the creation of cinemagraphs where specific regions of an image were animated.
Lumiere's unique design, emphasizing simultaneous spatial and temporal down-sampling and up-sampling, enabled extended video durations (up to 80 frames at 16 frames per second) with a single base model, contributing to improved computational efficiency and global temporal coherence. The model's versatility and ability to address challenges in large-scale T2V synthesis made it a promising tool for diverse content creation tasks. Experimental results and applications showcased Lumiere's effectiveness in generating coherent, realistic videos for various creative scenarios.
Evaluation and Comparisons
The Lumiere T2V model was evaluated on a dataset of 30 million videos with associated text captions, training a base model at 128x128 resolution with SSR output at 1024x1024 resolution. Performance was assessed on 113 diverse text prompts and further evaluated in a zero-shot scenario on the UCF101 dataset. Lumiere was compared against various T2V diffusion models, including ImagenVideo, AnimateDiff, StableVideoDiffusion, ZeroScope, Pika, and Gen2. Qualitative analysis revealed Lumiere's superiority in producing five-second videos with higher motion magnitude while maintaining temporal consistency.
Quantitative metrics, such as Frechet video distance (FVD) and inception score (IS), demonstrated competitive performance in zero-shot evaluation on UCF101. A user study, conducted through Amazon Mechanical Turk, supported Lumiere's preference over baselines in terms of visual quality, motion, and alignment with text prompts. The model's efficacy was highlighted in both qualitative and quantitative assessments, showcasing its advancements in large-scale T2V synthesis and superior performance compared to existing approaches.
Conclusion
In conclusion, the researchers introduced Lumiere, a T2V generation framework using a pre-trained T2I diffusion model. The proposed space-time U-Net architecture addressed limitations in coherent motion learning, presenting state-of-the-art results for various applications. However, the model currently can't handle multi-shot or scene transitions, posing avenues for future research. Lumiere's design principles can be extended to latent video diffusion models, suggesting potential advancements in T2V synthesis.
Video
Lumiere

*Important notice: arXiv publishes preliminary scientific reports that are not peer-reviewed and, therefore, should not be regarded as definitive, used to guide development decisions, or treated as established information in the field of artificial intelligence research.
Source:
Journal reference: