From intersections to roundabouts, GPD-1 brings unmatched accuracy to autonomous vehicle planning.
Study: GPD-1: Generative Pre-training for Driving. Image Credit: bigshot01 / Shutterstock

*Important notice: arXiv publishes preliminary scientific reports that are not peer-reviewed and, therefore, should not be regarded as definitive, used to guide development decisions, or treated as established information in the field of artificial intelligence research.
A recently posted on the arXiv preprint* server introduced "Generative Pre-training for Driving version 1 (GPD-1)," a novel framework designed to enhance the capabilities of autonomous driving systems. This approach models diverse driving scenarios to streamline tasks such as scene generation, motion planning, and traffic simulation, aiming to improve autonomous vehicles' ability to predict and simulate various driving conditions. GPD-1 leverages advanced innovations such as hierarchical positional tokenizers and vector-quantized autoencoders to achieve this integration.
Advancements in Autonomous Driving Technologies
In recent years, the field of autonomous driving has advanced significantly, primarily due to progress in artificial intelligence (AI) and machine learning technologies. Traditional methods often address specific aspects of driving scenarios, such as map generation or motion prediction, in isolation. However, integrating these components into a unified framework remains challenging because of the complexities involved in modeling interactions among various entities in a driving environment. Developing generative models, particularly autoregressive transformer architectures, offers promising solutions by effectively simulating and predicting driving scenarios using large datasets.
GPD-1: Development of a Unified Generative Model
In this paper, the authors introduced the GPD-1, a model designed to unify various aspects of scene evolution in autonomous driving. Their framework represents driving scenarios using tokens that encapsulate information about the ego vehicle, agents, and map elements. Leveraging an autoregressive transformer, it processes tokens sequentially, capturing temporal dependencies and spatial relationships to provide a robust representation of driving scenarios. The use of a scene-level attention mechanism facilitates nuanced interactions among tokens within each frame.
To encode spatial and temporal information, the researchers developed a hierarchical positional tokenizer for ego and agent tokens, incorporating two-dimensional (2D) positions and headings. For map tokens, a vector-quantized autoencoder (VQ-VAE) compresses ego-centric semantic maps into discrete tokens, effectively reducing complexity while maintaining spatial accuracy. This innovative design enables GPD-1 to generalize across tasks such as traffic simulation, scene generation, closed-loop simulation, map prediction, and motion planning without requiring additional fine-tuning.
The model was pre-trained on the extensive nuPlan dataset, which comprises over 1,300 hours of driving data collected from diverse urban environments, including complex scenarios such as intersections and roundabouts. This dataset provided a solid foundation for evaluating the model's performance in real-world scenarios.
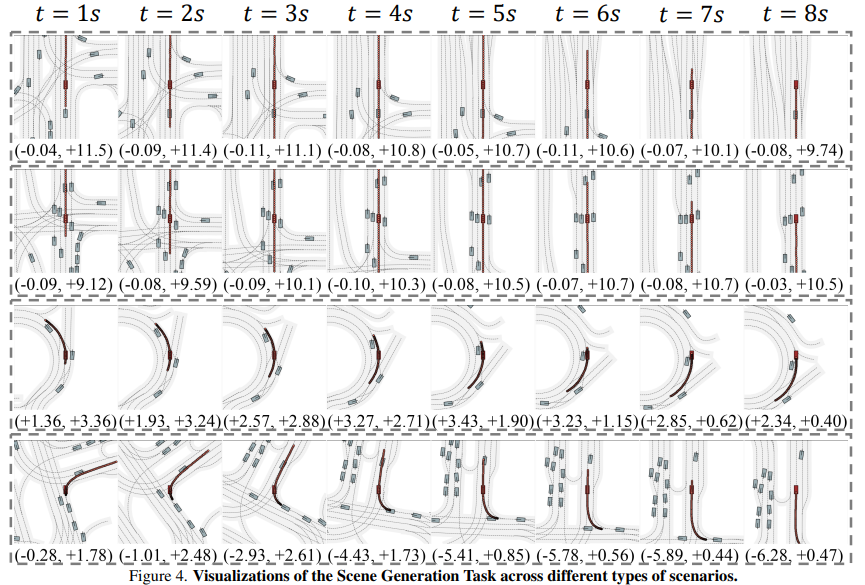
Figure shows the performance under the Scene Generation setting in complex scenarios. The results demonstrate that even in highly intricate road conditions, the map can be generated smoothly. In two turning scenarios, both the ego vehicle and agents follow a natural trajectory at a relatively steady speed. Similarly, in two straight-driving scenarios, the model effectively captures surrounding agents' actions (e.g., turning, driving, and decelerating) while maintaining a stable forward speed.
Key Findings and Insights
The study demonstrated the effectiveness of the GPD-1 model in generalizing across various autonomous driving tasks without requiring additional fine-tuning. In scene generation, it seamlessly initializes scenes by generating agent, map, and ego vehicle information. For traffic simulation, the model accurately predicted traffic evolution based on initial agent states and ground-truth maps, showing adaptability in dynamic environments.
Additionally, the model outperformed in motion planning, generating precise trajectories for the ego vehicle by leveraging information about surrounding agents and the map. Notably, GPD-1 achieved quantitative success, as measured by metrics such as Average Displacement Error (ADE), Final Displacement Error (FDE), and collision rates, showcasing its safety and precision. These outcomes showed that GPD-1 achieved state-of-the-art performance in multiple downstream tasks, particularly in motion planning, where it competes effectively with existing methods.
The experiments further revealed that GPD-1 maintained low collision rates during simulations, ensuring safety in dynamic driving scenarios. It exhibited robustness in managing interactions among multiple agents while following traffic rules and regulations. By accurately forecasting future scene evolutions and agent movements, GPD-1 represents a significant advancement in autonomous driving technology.
Practical Applications of GPD-1
The GPD-1 model has significant implications for advancing autonomous driving systems. Its ability to generate realistic driving scenarios from minimal initial inputs makes it a valuable tool for training and evaluating autonomous technologies.
In traffic simulation, GPD-1 effectively assesses vehicle interactions in complex environments, which can be valuable for researchers and developers in testing various driving conditions. Its motion planning capabilities provide a data-driven alternative to traditional methods, delivering precise trajectory predictions. Closed-loop simulations benefit from GPD-1’s ability to dynamically adapt agent trajectories based on real-time ego vehicle data, improving realism and safety.
The model also excels in closed-loop simulations by dynamically adapting agent trajectories based on real-time data from the ego vehicle. This adaptability can potentially enhance the safety and reliability of autonomous systems, especially in urban settings.
Conclusion and Future Directions
In conclusion, the GPD-1 model represents a significant step toward a fully integrated and interpretable framework for autonomous driving. By unifying diverse aspects of driving simulation into a cohesive system, it addresses the limitations of traditional approaches and provides a flexible, robust solution for realistic and adaptive driving simulations.
Future work should focus on enhancing the model's predictive accuracy in scenarios involving new agents entering the field of view, which has proven challenging due to their absence in the input data. Further fine-tuning on specialized datasets or incorporating adaptive learning mechanisms could improve its performance in dynamic, complex environments. Integrating real-time data processing and adaptive learning mechanisms could further improve its applicability in complex driving environments. Overall, the GPD-1 framework sets the foundation for advancing autonomous driving technologies, paving the way for continued innovation and significant contributions to the field.

*Important notice: arXiv publishes preliminary scientific reports that are not peer-reviewed and, therefore, should not be regarded as definitive, used to guide development decisions, or treated as established information in the field of artificial intelligence research.
Sources:
Journal reference:
- Preliminary scientific report.
Xie, Z., et al. GPD-1: Generative Pre-training for Driving. arXiv, 2024, 2412.08643. DOI: 10.48550/arXiv.2412.08643, https://arxiv.org/abs/2412.08643