AI-powered multi-scale solutions are reshaping how the chemical industry tackles decarbonization, promising a more efficient, sustainable, and low-carbon future—despite current deployment hurdles.
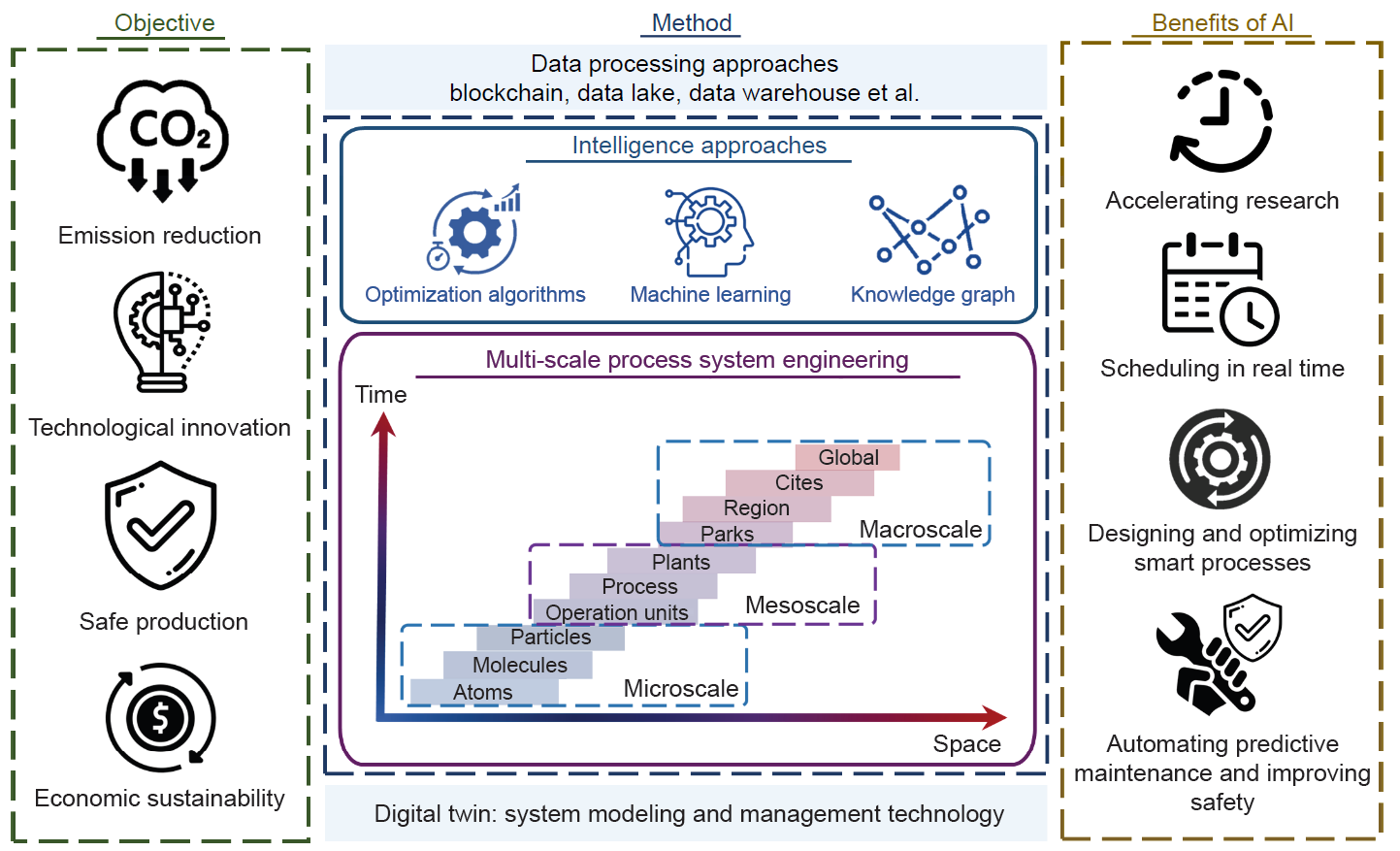
Empowering sustainable development: AI-enhanced multi-scale smart systems for the decarbonization of the chemical industry. Credit Technology Review for Carbon Neutrality, Tsinghua University Press
As the chemical industry seeks sustainable transformation, decarbonization requires intelligent solutions across multiple scales to enhance efficiency and reduce emissions. A research team led by Professor Xiaonan Wang at Tsinghua University has systematically reviewed AI-driven multi-scale smart systems for decarbonizing this energy-intensive sector.
Published in the journal Technology Review for Carbon Neutrality, the study explores innovations from materials discovery to industrial park optimization, highlighting the role of cross-scale modeling in addressing complex chemical processes. The findings provide insights for policymakers and industry leaders to advance smart, sustainable, and carbon-neutral chemical production.
The review highlights the role of AI in simplifying traditional mechanistic models, improving efficiency, and promoting resource conservation. At the microscopic scale, machine learning aids in material design and performance prediction, such as catalysts for CO₂ conversion and membrane optimization in separation processes, with emerging research focusing on uncovering underlying mechanisms. However, the paper also emphasizes that many of these developments remain in the pilot or conceptual stage, with industrial deployment still limited. Data reliability remains a major challenge.
At the mesoscale, AI-driven process modeling using surrogate models and hybrid machine learning-physical frameworks accelerates industrial applications of decarbonization technologies, with the integration of digital twins significantly reducing computational time. However, scaling up digital integration remains a key hurdle.
At the macro level, industrial symbiosis strategies optimize chemical parks by linking production with external markets and environmental factors, supported by digital infrastructure that enables dynamic coordination of resources and emissions, with digital twin technology enabling real-time operational adjustments. These twins also provide a feedback mechanism for continuous improvement by integrating operational data directly into predictive models.
Despite promising advancements, the application of intelligent technologies in the chemical industry remains largely in the research phase. The review stresses that deployment at an industrial scale is hampered by the need to harmonize technologies across physical and digital layers. Cross-scale modeling is essential for bridging molecular-scale innovations with industrial-scale applications, while AI-driven optimization enhances efficiency and sustainability. These models span from atomic and nanoscale phenomena to full-plant logistics, requiring seamless integration across spatial and temporal domains.
However, full-scale industrial implementation faces challenges spanning technical, economic, social, and ethical dimensions. These include data security, infrastructure compatibility, AI interpretability and algorithmic transparency, workforce displacement due to automation-driven transitions, integration into emerging carbon pricing mechanisms, and regulatory considerations. Notably, the authors highlight the need for transparent, interpretable AI models and raise concerns about workforce transitions as automation expands.
The study underscores the need for interdisciplinary collaboration across materials science, carbon accounting, and supply chain logistics, and multi-stakeholder cooperation to address these challenges and accelerate the chemical industry's transition to carbon neutrality. By integrating AI and digital technologies across scales, the industry can advance toward a more efficient, sustainable, and low-carbon future. The authors argue that digital twins, hybrid modeling approaches, and active learning algorithms will be foundational in enabling this transformation, especially as real-world applications move beyond simulation environments.
This work is supported by the National Key R&D Program of China (2023YFE0204600), the Tsinghua University Initiative Scientific Research Program, and the Special Project of the National Natural Science Foundation (No. 42341204).
Source:
Journal reference: