Unlike conventional AI models trained on synthetic edits, REALEDIT captures real user modifications, making AI-powered photo editing more accurate, practical, and aligned with real-world needs.
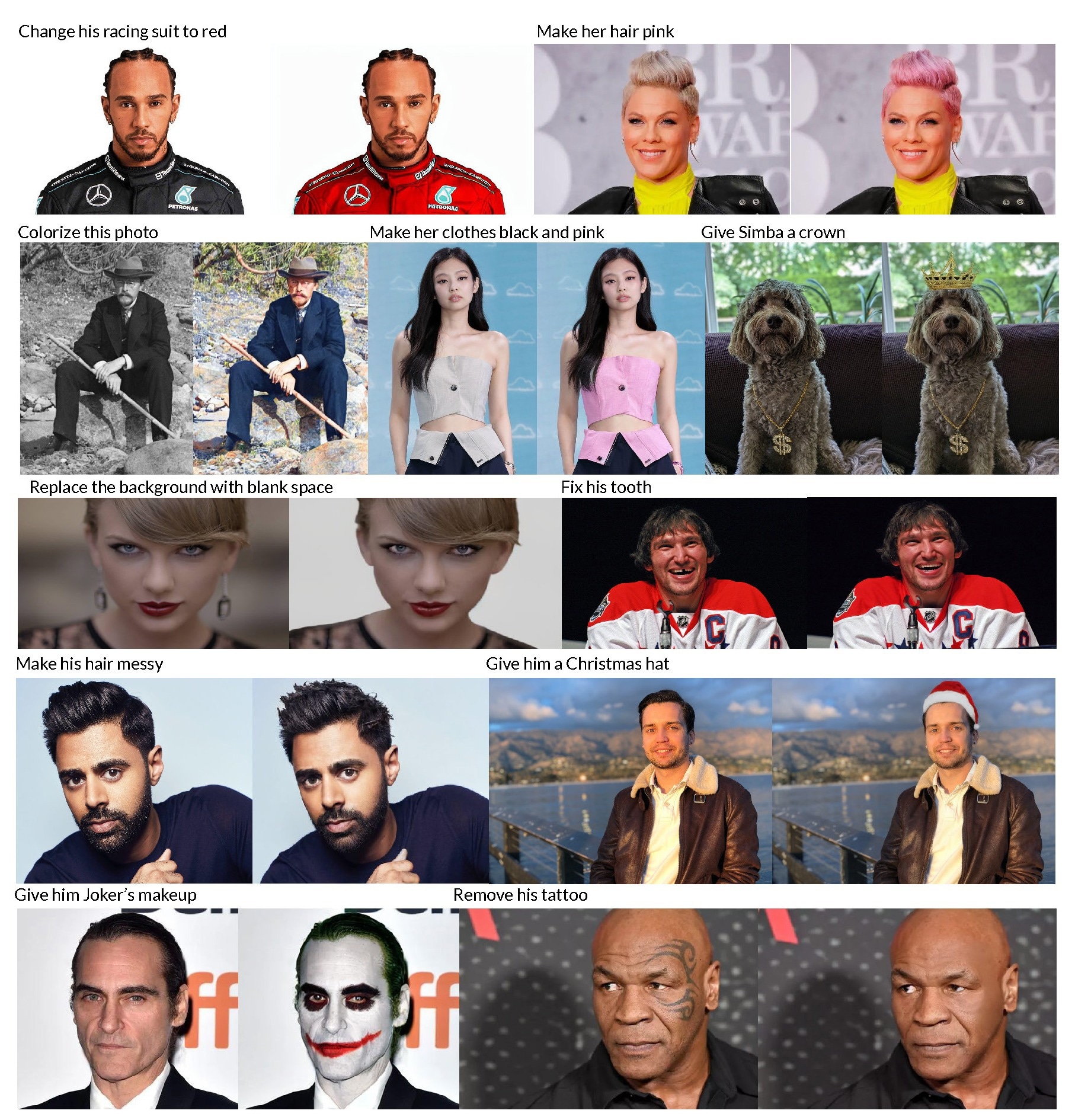
We visualize edits made by our model. We introduce REALEDIT, a large-scale image editing dataset sourced from Reddit with real-world user edit requests and human-edits. By finetuning on REALEDIT, our resultant model outperforms existing models by up to 165 Elo points with human judgment and delivers real world utility to real user requests online.

*Important notice: arXiv publishes preliminary scientific reports that are not peer-reviewed and, therefore, should not be regarded as definitive, used to guide development decisions, or treated as established information in the field of artificial intelligence research.
Image editing has become an essential tool for personal and professional use, with millions of people making digital modifications to their photos daily. Despite advancements in artificial intelligence, most AI-driven image editing models fail to meet real-world user expectations.
While existing models perform well on controlled benchmarks, they struggle with common requests such as removing objects, enhancing clarity, or restoring damaged photos. This limitation largely stems from the datasets used to train them, which rely on artificial transformations rather than edits that reflect practical user needs.
To address this gap, a research team from the University of Washington introduced REALEDIT, a large-scale dataset compiled from Reddit’s image-editing communities. Unlike conventional datasets, which primarily feature synthetic modifications, REALEDIT consists of over 57,000 real user requests and their corresponding human-made edits. The paper is published on the arXiv preprint* server.
A key feature of REALEDIT is its rigorous multi-stage verification process, where AI moderation tools and manual review ensure only high-quality, appropriate edits are included. By fine-tuning an AI model with this dataset, the researchers achieved substantial performance improvements, with their model outperforming competitors by 165 Elo points in human preference rankings and showing a 92% increase in automated evaluation metrics.
However, while REALEDIT excelled in real-world tests, its performance on synthetic benchmarks such as GenAI Arena was competitive but not always superior to existing models. The success of this model underscores the importance of training on real-world data rather than artificially generated examples.
The Limitations of Current Image Editing Models
Despite the progress in AI-generated imagery, existing models continue to struggle with practical image-editing tasks. Platforms like Reddit’s r/PhotoshopRequest and r/estoration, where users request and execute real-world edits, reveal a broad spectrum of needs. People seek to remove distracting objects, adjust colors, fix lighting, and restore old photographs. Many even offer payment for high-quality edits, further demonstrating the demand for reliable image-editing solutions.
Current datasets fail to reflect these real-world editing requirements. They often include tasks such as inserting random objects or making artistic modifications, which do not align with typical user needs. Ultra-Edit, one of the largest datasets used for training AI editing models, primarily consists of artificial alterations, making it unsuitable for handling common tasks like object removal or enhancement.
This gap leads to models that struggle with fundamental real-world requests, as synthetic datasets tend to focus more on adding elements rather than refining or correcting existing images. Models trained on such datasets struggle when applied to real-world requests, reinforcing the necessity for a dataset like REALEDIT that represents actual user demands.
Constructing the REALEDIT Dataset
The researchers developed a meticulous process to build a dataset that accurately captures the diversity of real-world image edits. They collected over 261,000 posts and 1.1 million comments from Reddit’s r/PhotoshopRequest and r/estoration communities, spanning nearly a decade of user-submitted content. Recognizing that many user requests include personal anecdotes or unnecessary details, the team used GPT-4o to refine the instructions into clear, actionable editing directives, ensuring they were concise and relevant.
A rigorous manual verification process ensured that only high-quality examples were included in the dataset. Images containing inappropriate or ambiguous edits were removed, resulting in a final dataset of 48,000 training examples and 9,300 test examples. The dataset was also filtered to align with the pretraining distribution of InstructPix2Pix, ensuring that AI models trained on REALEDIT would perform well while maintaining a structured, human-centered approach to editing. Unlike previous datasets, REALEDIT includes genuine user edits, which allows AI models trained on it to perform significantly better in real-world applications.
Evaluating Image Editing Models on Real-World Data
To assess the effectiveness of AI-driven image editing, the researchers fine-tuned InstructPix2Pix, a leading text-guided editing model, using the REALEDIT dataset. To improve the model’s ability to handle human-centric edits, they replaced its Stable Diffusion decoder with OpenAI’s Consistency Decoder, which significantly enhances fine-grained details such as facial features, text clarity, and texture precision.
The model was tested against six state-of-the-art image editing models, including MagicBrush, AURORA, HIVE, and SDEdit. Evaluation was conducted using both automated metrics and human preference rankings. The REALEDIT model outperformed all competitors, achieving a 4.61 score on the VIEScore metric, compared to 2.4 for the second-best model. However, on synthetic datasets such as GenAI Arena, the model performed competitively rather than outright dominating, indicating that training on real-world data may not always generalize perfectly to artificially curated benchmarks.
To validate its real-world usability, the researchers deployed the model on Reddit and provided free editing services to users. The responses were overwhelmingly positive. One user, who requested red-eye removal, commented, “Thank you so much! Solved.” Another user, who needed a background object removed, was impressed with the result, saying, “Wow, this looks great! I love the way you smoothed out the lighting on me as well.” These real-world tests provided an additional layer of validation, confirming that models trained on REALEDIT offer practical value beyond controlled laboratory benchmarks.
Enhancing Deepfake Detection with REALEDIT
Beyond image editing, REALEDIT has significant implications for the detection of manipulated images. The researchers partnered with a deepfake detection non-profit to explore how the dataset could improve AI’s ability to distinguish between real and altered photos. Many existing deepfake detection models are trained on digitally generated fakes but struggle with detecting human-edited images, which are often more subtle and sophisticated.
By incorporating REALEDIT into the training process, the researchers enhanced a Universal Fake Detect (UFD) model’s ability to identify manipulated images. Initially, the baseline model had an F1-score of 23.5, but after fine-tuning with REALEDIT, its score improved to 69—a dramatic 45.5-point increase. When tested on a separate dataset of in-the-wild edited images, the model’s performance rose from 49 to 63, demonstrating the dataset’s effectiveness in improving deepfake detection capabilities.
Ethical Considerations and Privacy Safeguards
Since REALEDIT is sourced from publicly available Reddit posts, privacy and ethical considerations were carefully addressed. The dataset does not store images directly but instead references their original URLs. If a user deletes an image from Reddit, it is automatically removed from REALEDIT. Additionally, users can request the removal of their data via an online form.
To prevent inappropriate use, the dataset was filtered using AI moderation tools and manual review. Explicit or unethical content was excluded, ensuring that the dataset remains a safe and ethical resource for AI training. Despite these safeguards, the researchers acknowledge that Reddit’s user base is predominantly Western, young, and male, which may introduce demographic biases into the dataset. Future efforts will focus on expanding the dataset’s diversity to better reflect a broader range of user needs and cultural contexts.
Transforming Image Editing and AI Research
REALEDIT represents a groundbreaking shift in AI-driven image editing by replacing artificial training data with real-world user requests and edits. The dataset enables models to better align with human expectations, leading to significant improvements in both automated benchmarks and human evaluations. The success of the REALEDIT-trained model in real-world applications, as demonstrated by positive user feedback on Reddit, underscores its practical utility.
Beyond editing, REALEDIT’s impact extends to deepfake detection, where it has been shown to enhance AI’s ability to identify manipulated images. While the dataset has already demonstrated substantial benefits, future research will focus on improving its adaptability to both real-world and synthetic benchmarks, ensuring that AI models trained on it remain versatile and effective across diverse use cases.
By continuing to refine and expand the dataset, researchers can further improve AI-driven editing tools, making them more accessible and effective for everyday users. The findings of this study highlight the importance of training AI on authentic human-generated data, paving the way for future advancements in AI-powered image editing and content verification.

*Important notice: arXiv publishes preliminary scientific reports that are not peer-reviewed and, therefore, should not be regarded as definitive, used to guide development decisions, or treated as established information in the field of artificial intelligence research.
Journal reference:
- Preliminary scientific report.
Sushko, P., Bharadwaj, A., Lim, Z. Y., Ilin, V., Caffee, B., Chen, D., Salehi, M., Hsieh, C., & Krishna, R. (2025). REALEDIT: Reddit Edits As a Large-scale Empirical Dataset for Image Transformations. ArXiv. https://arxiv.org/abs/2502.03629