Breaking barriers in communication: a new AI-driven neural network deciphers sign languages with unprecedented precision, paving the way for inclusive interactions worldwide.
Research: Word-Level Sign Language Recognition With Multi-Stream Neural Networks Focusing on Local Regions and Skeletal Information. Image Credit: Andrey_Popov / Shutterstock
Nations worldwide have developed sign languages to fit their local communication styles. Each language consists of thousands of signs, making it difficult to learn and understand. However, the work of an Osaka Metropolitan University-led research group has now improved the accuracy of sign language recognition by using artificial intelligence to translate signs into words automatically. The research, published in the journal IEEE Access, has been able to improve the accuracy of sign language recognition.
The researchers developed a novel multi-stream neural network (MSNN) to enhance recognition accuracy. This system combines global movement analysis with localized hand, face, and skeletal position data to better understand signs. Previous research methods focused on capturing information about the signer's general movements. Accuracy problems have stemmed from the different meanings that could arise based on subtle differences in hand shape and the relationship between the hands and the body.
Associate Professor Katsufumi Inoue and Associate Professor Masakazu Iwamura of the Graduate School of Informatics worked with colleagues, including at the Indian Institute of Technology Roorkee, to improve AI recognition accuracy. They added data on the general movements of the signer's upper body, such as hand and facial expressions, as well as skeletal information on the position of the hands relative to the body.
The research team tested their method on two major datasets, WLASL and MS-ASL, which are widely used for American Sign Language (ASL) recognition. Their model achieved Top-1 accuracy improvements of approximately 10–15% compared to conventional methods. For example, it achieved 81.38% accuracy on the WLASL100 dataset.
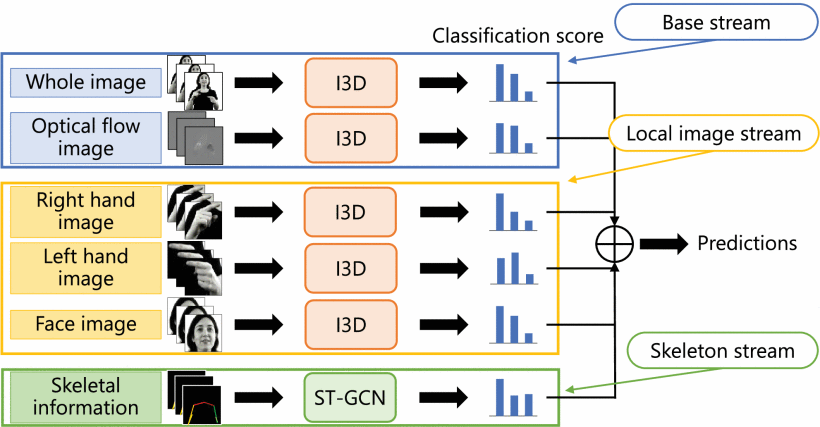
Overview of the proposed method. The proposed multi-stream neural network (MSNN) consists of three streams: 1) a base stream, 2) local image stream, and 3) skeleton stream. Each stream is trained separately, and the recognition scores extracted from each stream are averaged to obtain the final recognition result.
"We were able to improve the accuracy of word-level sign language recognition by 10-15% compared to conventional methods," Professor Inoue declared. "Our method uses streams for global movement, localized hand and facial features, and skeletal data, which allows us to capture subtle distinctions in gestures. In addition, we expect that the proposed method can be applied to any sign language, hopefully leading to improved communication with speaking- and hearing-impaired people in various countries."
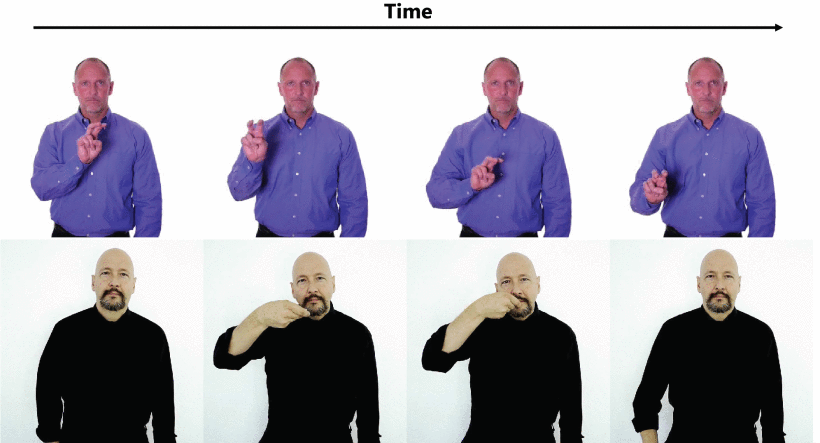
Examples of different gestures that represent the word “pizza” from the WLASL dataset
The study also identified challenges in recognizing visually similar signs and handling diverse data from different environments, such as varying viewpoints and complex backgrounds. Future work will aim to improve the method’s scalability, enhance robustness for real-world applications, and explore its adaptability to sign languages other than ASL, such as British, Japanese, and Indian sign languages.
Source:
Journal reference:
- M. Maruyama, S. Singh, K. Inoue, P. Pratim Roy, M. Iwamura and M. Yoshioka, "Word-Level Sign Language Recognition With Multi-Stream Neural Networks Focusing on Local Regions and Skeletal Information," in IEEE Access, vol. 12, pp. 167333-167346, 2024, doi: 10.1109/ACCESS.2024.3494878, https://ieeexplore.ieee.org/document/10749796