Study reveals how biased generative AI systems evade current testing methods, urging actionable solutions to align fairness assessments with real-world regulatory demands.
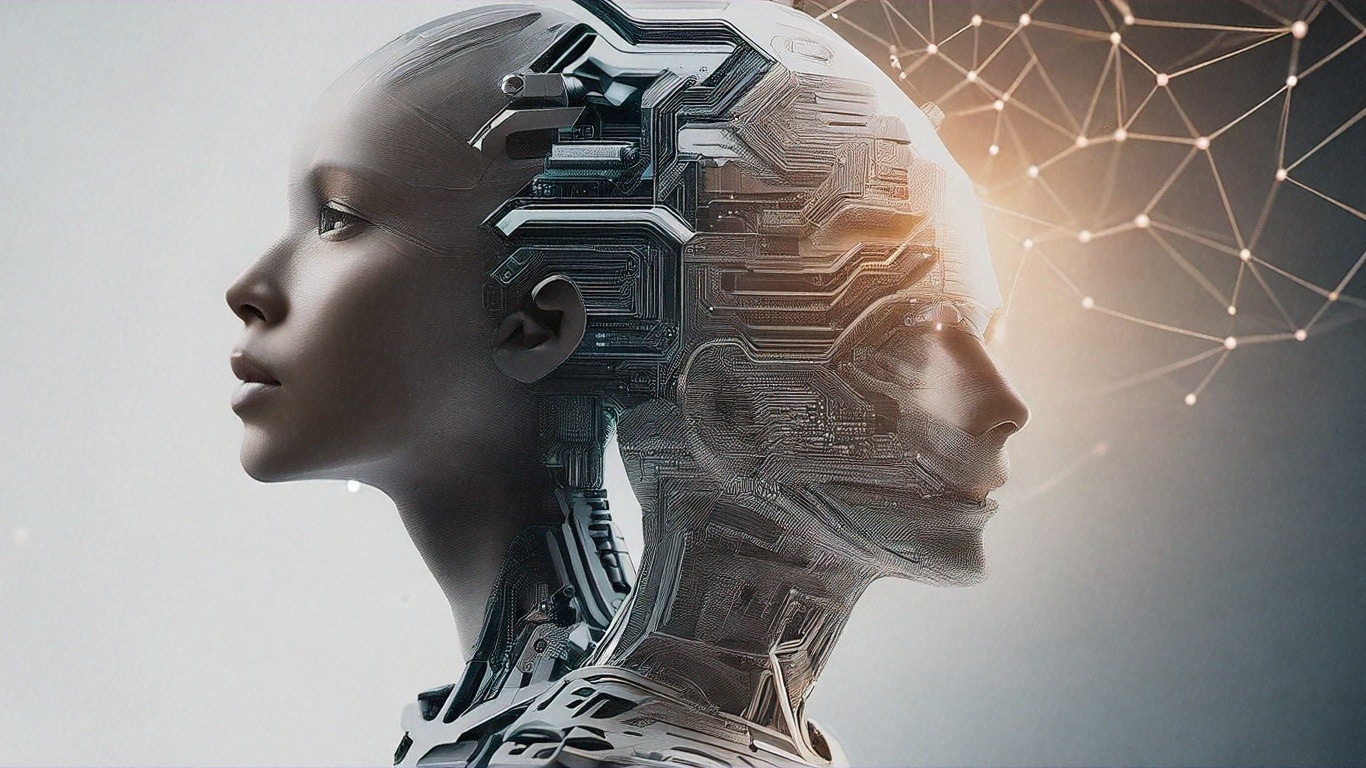
Research: Towards Effective Discrimination Testing for Generative AI

*Important notice: arXiv publishes preliminary scientific reports that are not peer-reviewed and, therefore, should not be regarded as definitive, used to guide development decisions, or treated as established information in the field of artificial intelligence research.
In an article submitted to the arXiv preprint* server, Columbia University and New York University researchers addressed the challenges of regulating discriminatory behavior in generative artificial intelligence (GenAI) models. They argued that fairness research has not effectively bridged the gap between biased assessment methods and regulatory goals, leading to flawed evaluations and potentially discriminatory deployments.
Through four case studies, they highlighted how misalignment between fairness testing techniques and regulations results in adverse real-world outcomes. The case studies revealed issues such as biased resume summaries, the variability of fairness metrics, and the impact of multi-turn interactions and user modifications, which complicate regulatory efforts. They proposed practical improvements to discrimination testing to align better with regulatory standards and ensure reliable fairness assessments.
Related Work
Past studies revealed discriminatory behaviors in GenAI, including biased outputs, underrepresentation in prompts, and performance drops with minority dialects.
Researchers highlighted the lack of standardized discrimination measures and the instability of fairness testing due to factors like distribution shifts. This instability increases risks of manipulation, or "d-hacking," where tests show low bias without reflecting real-world behavior.
For example, small changes in evaluation setups—such as the choice of testing frameworks or metrics—can produce misleading results about a model's fairness. Recent work emphasized regulatory gaps and the need for robust bias assessment to ensure fairness in GenAI systems.
Regulating GenAI Fairness
Emerging regulatory approaches to address fairness and discrimination in GenAI fall into two categories: applying traditional discrimination laws and creating AI-specific frameworks.
Traditional U.S. laws like Title VII and the Equal Credit Opportunity Act (ECOA) rely on doctrines such as disparate treatment and disparate impact to prevent both direct and indirect discrimination in decisions like hiring or credit approvals.
While existing laws can apply to GenAI systems making allocative decisions, they often fail to capture concerns like representational harms or toxic content generation. For instance, text-to-image models may generate over-sexualized portrayals of women from certain demographic groups, an issue outside the scope of traditional anti-discrimination laws. It has led to new AI-focused regulations, such as the EU AI Act and the AI Bill of Rights, emphasizing fairness assessments, adversarial testing, and liability distribution between developers and deployers. However, challenges remain, such as ambiguity around liability and the need for comprehensive fairness evaluations.
Despite progress, significant misalignments exist between regulatory goals and current fairness testing methods for GenAI. Legal frameworks often lack clear metrics and testing protocols, leaving room for discretionary fairness tests that may comply with regulations but fail to address real-world discriminatory risks.
The paper notes that current fairness testing methods often overlook complexities such as multi-turn interactions, adaptive deployments, and user-driven parameter changes, which are critical for real-world GenAI systems.
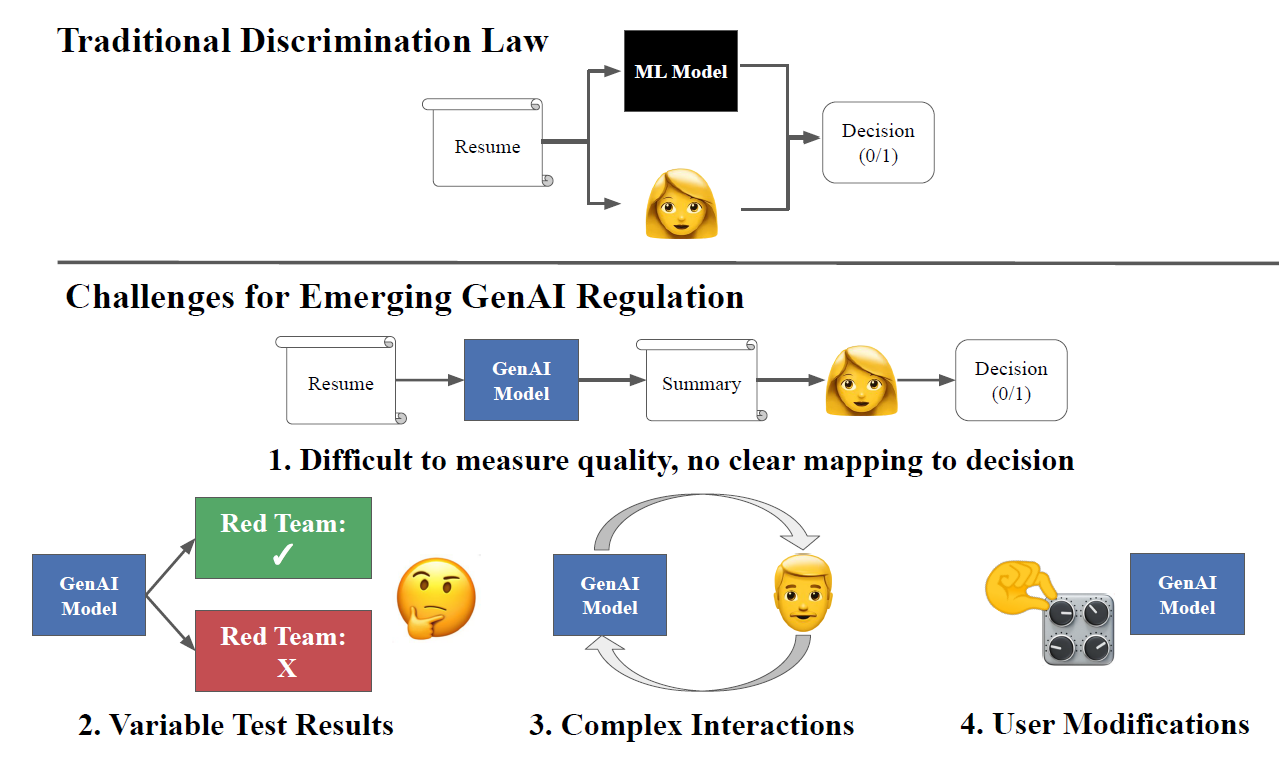
The output of classification models can often be directly mapped onto allocative decisions, and thus, traditional discrimination law can be applied directly. GenAI models bring unique challenges to applying both existing and emerging regulations. Most notably: 1) outputs are difficult to evaluate and do not clearly map onto decisions; 2) complex interaction modes, such as multi-turn dialogue, cannot be easily recreated in test settings; 3) testing procedures (e.g., a particular red teaming approach) are sensitive to small changes in conditions and give highly variable results; 4) users may modify models after deployment, for example by changing sampling parameters.
Challenges in GenAI Fairness
The report case studies highlight gaps between traditional fairness evaluation methods and their alignment with regulatory goals in GenAI systems, particularly in discrimination testing.
The first case study focuses on resume screening, using an experimental setup in which large language models (LLMs) summarize resumes with race-associated names. Popular evaluation metrics like recall-oriented understudy for gisting evaluation (ROUGE) often overlook deeper biases, leading to scenarios where models deemed fair by these metrics result in discriminatory downstream outcomes.
In the study, Llama-2-7B, chosen for its high ROUGE score, exhibited disparities in interview selection rates, favoring white candidates over Black and Hispanic candidates.
Further analysis indicated that race-biased summarization, rather than decision-making, was the primary source of discrimination. Mitigation strategies suggested designing fairness metrics that evaluate downstream effects rather than surface-level qualities. For instance, metrics assessing descriptive language, tone, or content differences across demographics could better capture biases.
The authors demonstrated the potential of tailored evaluation suites incorporating sentiment, summary length, and emotional intelligence indicators to identify less discriminatory models like Gemma-2-2B and align fairness and task performance.
These findings underscore the need for context-aware evaluation frameworks to ensure equitable AI outcomes and actionable policy development.
Additionally, the variability in fairness testing results—driven by different red teaming models or testing configurations—underscores the importance of standardizing testing methods. This would reduce inconsistencies in evaluating GenAI systems under diverse conditions.
Conclusion
To sum up, the proposed research shift focused on developing context-specific, robust testing frameworks to address fairness in GenAI systems. While case studies highlighted key challenges, they did not cover the full spectrum of real-world issues, such as prompt sensitivity or model interpretability.
The authors emphasized the need for further research to address dynamic fairness assessment, evolving models, and additional deployment scenarios. Future work was encouraged to explore evolving models and dynamic fairness assessment.

*Important notice: arXiv publishes preliminary scientific reports that are not peer-reviewed and, therefore, should not be regarded as definitive, used to guide development decisions, or treated as established information in the field of artificial intelligence research.
Journal reference:
- Preliminary scientific report.
Zollo, T. P., et al. (2024). Towards Effective Discrimination Testing for Generative AI. ArXiv. DOI: 10.48550/arXiv.2412.21052, https://arxiv.org/abs/2412.21052