Researchers uncover how human-AI interactions don’t just mirror biases—they magnify them, reshaping decisions and perceptions in profound ways.
Research: How human–AI feedback loops alter human perceptual, emotional and social judgements. Image Credit: Suri_Studio / Shutterstock
In an article recently published in the journal Nature Human Behaviour, researchers at the University College London, UK, and Massachusetts Institute of Technology, USA, investigated how human-artificial intelligence (AI) interactions amplified biases in human perceptual, emotional, and social judgments.
Through experiments with 1,401 participants and various tasks, the authors revealed a feedback loop where AI systems not only amplified biases but also influenced humans to internalize these biases, leading to a snowball effect of escalating errors in judgment. For example, in one task, biases increased by over 10% after repeated interactions with biased AI.
The study highlighted the significant and often unrecognized influence of AI systems compared to human-only interactions.
Background
Human–AI interactions are becoming increasingly prevalent, influencing decision-making in domains like healthcare, education, and finance. Modern AI systems, powered by machine learning techniques like convolutional neural networks (CNNs) and transformers, identify patterns in large datasets to assist human tasks. However, these systems often perpetuate or amplify biases inherent in their training data, such as racial or gender biases, which can propagate to humans interacting with them.
Previous research has focused on how biases arise within AI systems and their societal implications. Studies have shown that biased datasets lead to biased AI outputs. Still, little attention has been paid to the reverse effect: how biased AI systems alter human cognition and amplify existing human biases.
The researchers emphasize that biases are amplified because humans perceive AI as less noisy and more reliable than other humans, making them more susceptible to AI influence. This gap in understanding limits efforts to mitigate AI-related bias amplification.
This paper addressed this gap by empirically demonstrating how human-AI interactions amplified perceptual, emotional, and social biases, uncovering a feedback loop where biases escalated over time.
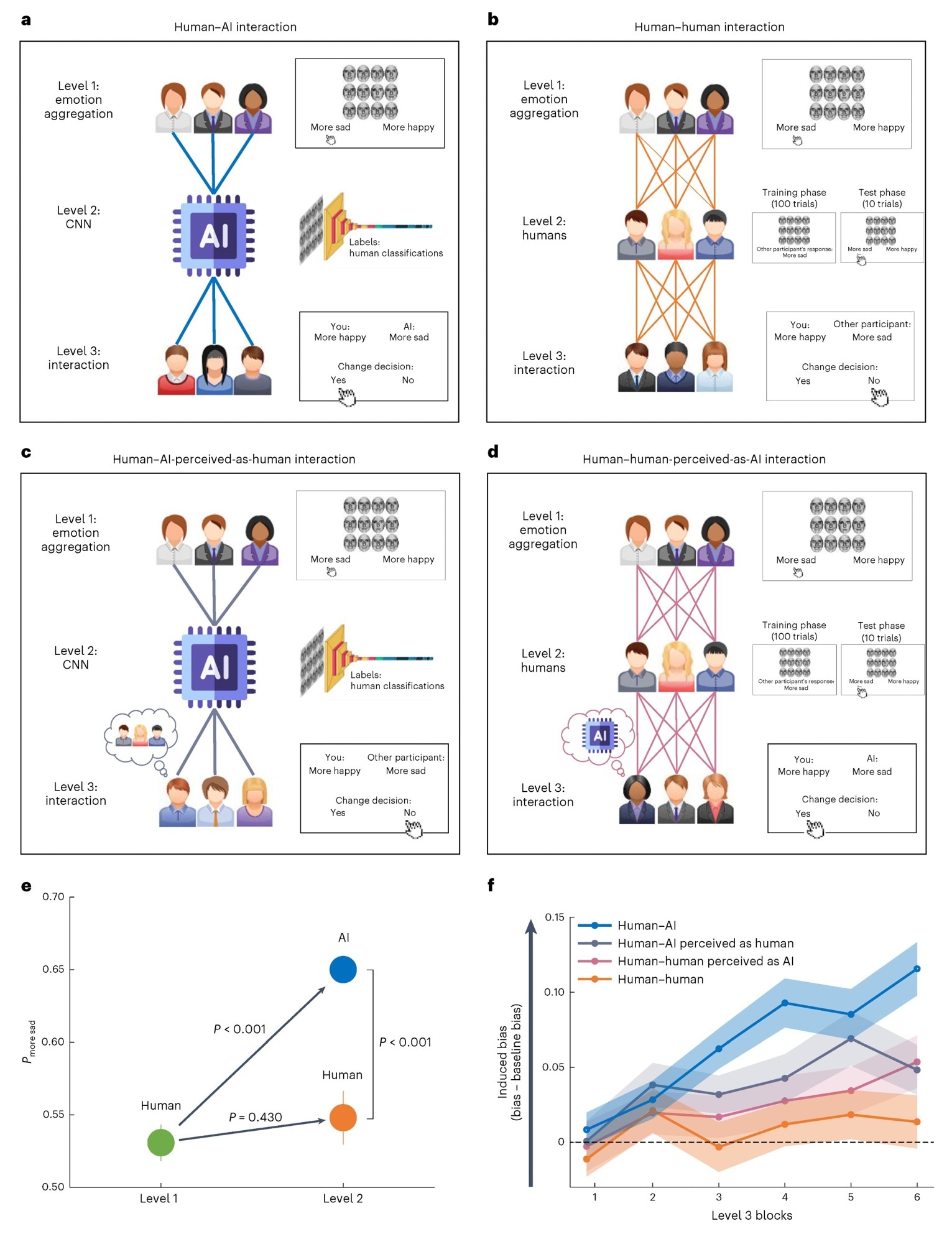
Human–AI interaction creates a feedback loop that makes humans more biased (experiment 1). a, Human–AI interaction. Human classifications in an emotion aggregation task are collected (level 1) and fed to an AI algorithm (CNN; level 2). A new pool of human participants (level 3) then interact with the AI. During level 1 (emotion aggregation), participants are presented with an array of 12 faces and asked to classify the mean emotion expressed by the faces as more sad or more happy. During level 2 (CNN), the CNN is trained on human data from level 1. During level 3 (human–AI interaction), a new group of participants provide their emotion aggregation response and are then presented with the response of an AI before being asked whether they would like to change their initial response. b, Human–human interaction. This is conceptually similar to the human–AI interaction, except the AI (level 2) is replaced with human participants. The participants in level 2 are presented with the arrays and responses of the participants in level 1 (training phase) and then judge new arrays on their own as either more sad or more happy (test phase). The participants in level 3 are then presented with the responses of the human participants from level 2 and asked whether they would like to change their initial response. c, Human–AI-perceivedas- human interaction. This condition is also conceptually similar to the human–AI interaction condition, except participants in level 3 are told they are interacting with another human when in fact they are interacting with an AI system (input: AI; label: human). d, Human–human-perceived-as-AI interaction. This condition is similar to the human–human interaction condition, except that participants in level 3 are told they are interacting with AI when in fact they are interacting with other humans (input: human; label: AI). e, Level 1 and 2 results. Participants in level 1 (green circle; n = 50) showed a slight bias towards the response more sad. This bias was amplified by AI in level 2 (blue circle), but not by human participants in level 2 (orange circle; n = 50). The P values were derived using permutation tests. All significant P values remained significant after applying Benjamini–Hochberg false discovery rate correction at α = 0.05. f, Level 3 results. When interacting with the biased AI, participants became more biased over time (human–AI interaction; blue line). In contrast, no bias amplification was observed when interacting with humans (human–human interaction; orange line). When interacting with an AI labeled as human (human–AI-perceived-as-human interaction; grey line) or humans labeled as AI (human–AI-perceived-as-human interaction; pink line), participants’ bias increased but less than for the human-AI interaction (n = 200 participants). The shaded areas and error bars represent s.e.m.
Experimental Methods
The authors investigated human-AI and human-human interactions in decision-making tasks, focusing on emotional aggregation and motion perception. Ethical approval was obtained, and all participants provided informed consent. A total of 1,401 participants, recruited via Prolific, completed experiments designed using PsychoPy3 and hosted on Pavlovia.
In the emotional aggregation task, participants evaluated arrays of morphed emotional faces, judging whether they were predominantly happy or sad. Variations of this task included interactions with AI and human associates and situations where participants were misled about the nature of their interaction (that is, AI perceived as human or vice versa).
For each scenario, response biases and accuracy were analyzed. Participants interacting with AI or human associates demonstrated different decision-making patterns, influenced by the perceived nature of the associate and prior biases.
In the random dot kinematogram (RDK) task, participants estimated the percentage of moving dots, evaluated their confidence, and interacted with AI algorithms (accurate, biased, or noisy). Participants assigned weights to their own and AI responses, affecting joint decision-making.
Results from this task revealed that biased AI significantly increased human bias over repeated interactions, whereas accurate AI improved accuracy. The order of algorithm interaction was randomized, and participants were surveyed on each algorithm's perceived influence and accuracy.
Another experiment used Stable Diffusion, a generative AI system, to assess real-world implications. Participants exposed to AI-generated images of financial managers—over-representing white men—were more likely to select white men as financial managers in subsequent judgments compared to those exposed to neutral images.
Across tasks, biases were assessed through relative decision changes, while accuracy improvements or declines were quantified. Results highlighted the nuanced impact of AI behavior (accuracy, bias, or noise) and human perceptions on collaborative decision-making processes.
Key Findings
The authors explored how human-AI interactions amplified biases, with critical findings across several experiments. When humans interacted with biased AI, their biases increased over time, unlike human-only interactions, where bias levels remained consistent.
For example, in an emotion classification task, AI trained on slightly biased data amplified the bias significantly, influencing human participants to further increase their bias. This feedback loop highlighted how human-AI collaboration could exacerbate bias compared to human-human interactions, which did not show such effects.
AI trained on biased data demonstrated a tendency to amplify bias beyond its initial levels, especially when noisy data was involved. Conversely, accurate AI improved human accuracy over time, suggesting the dual-edged potential of AI influence. For instance, in the RDK task, participants interacting with biased AI displayed a bias increase of over 5%, while those with accurate AI improved their judgment accuracy by a similar margin.
Participants interacting with biased AI were more likely to classify neutral stimuli in line with the AI’s bias, showing a progressive alignment with the AI's flawed patterns.
The experiments also revealed that participants underestimated the extent of AI's influence despite measurable shifts in their decisions. This underestimation, coupled with humans’ perception of AI as more reliable than other humans, explains why biases amplify faster during human-AI interactions.
Conclusion
In conclusion, the researchers demonstrated that human-AI interactions could amplify biases in human judgments, creating a feedback loop where biases grew over time.
The study revealed that when AI systems trained on biased data interact with humans, they not only reinforced the biases inherent in the AI but also influenced human participants to adopt these biases.
The impact of this feedback loop was observed in tasks involving emotion classification, motion perception, and social biases. Significantly, participants underestimated the influence of AI, making them more susceptible to its biases. Real-world experiments using generative AI further underscored this phenomenon, with biased outputs shaping social judgments.
The study highlighted the need for AI developers to address this issue, as biased AI systems could perpetuate and escalate biases in human decision-making. Furthermore, accurate AI systems improved human judgments, suggesting that reducing bias in AI could enhance decision-making across various domains, from hiring to healthcare.
The researchers propose strategies such as raising awareness about AI-induced biases and promoting transparency in AI design to mitigate these effects.