Discover how INTELLECT-1 redefines AI training with a decentralized, community-driven approach that bridges continents and democratizes advanced AI development.
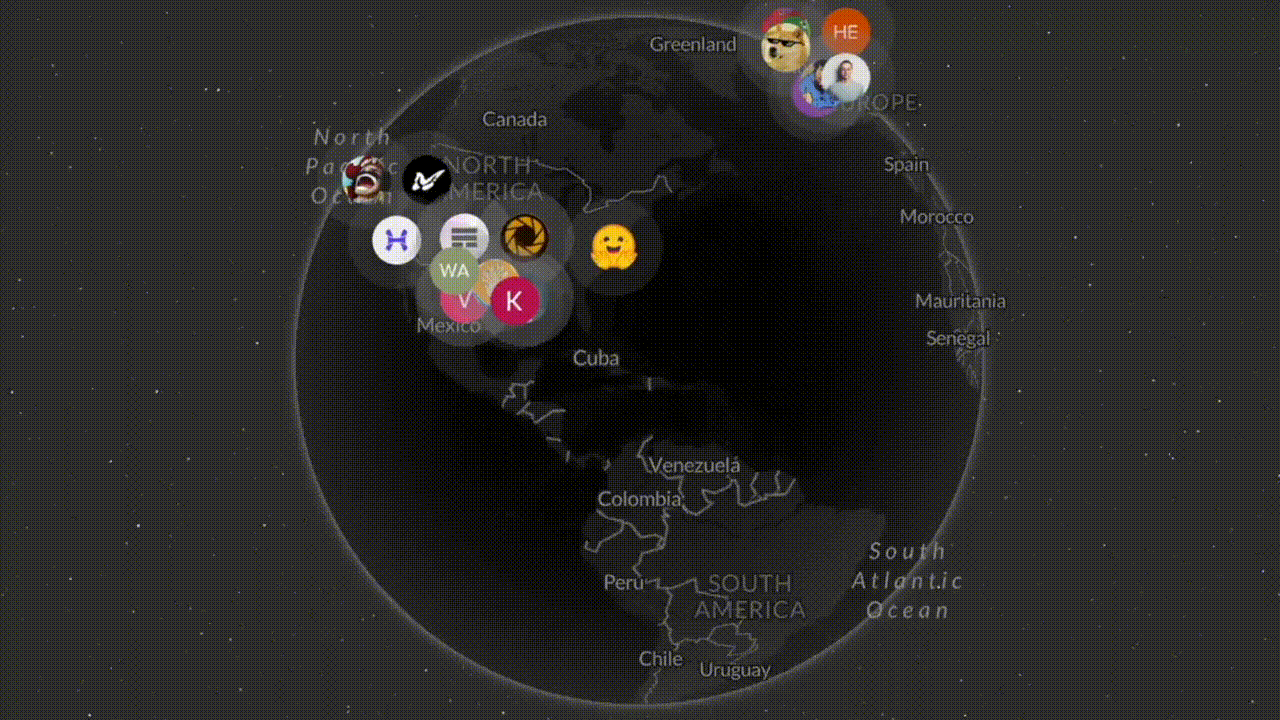
Image Credit: Primeintellect.ai
A recent article posted to the Primeintellect.ai website presented the first large-scale experiment in collaboratively training a 10 billion parameter model over one trillion tokens across five countries and three continents using up to 112 graphics processing units (GPUs).
The study demonstrated high compute utilization and minimal overhead compared to centralized training. Key innovations in the PRIME framework, such as ElasticDeviceMesh, live checkpoint recovery, and hybrid distributed local communication fully sharded data parallel (DiLoCo-FSDP2), significantly reduced communication bandwidth while ensuring training stability.
The work emphasized the potential of decentralized artificial intelligence (AI) development for democratizing future AI advancements, shifting the focus from corporate exclusivity to community-driven innovation. This milestone aligns with the broader mission of preventing the concentration of AI capabilities within a small number of organizations, promoting global collaboration instead.
Related Work
Past work in large-scale model training was primarily focused on centralized approaches, limiting the ability to scale globally. Previous research also highlighted the challenges of bandwidth constraints and node volatility in decentralized settings. However, innovations in distributed training, such as the PRIME framework, a custom int8 all-reduce kernel, and DiLoCo-FSDP2, paved the way for scalable, community-driven training of frontier models.
Revolutionizing Decentralized AI Training
INTELLECT-1 has been released as the first 10 billion parameter language model to be collaboratively trained globally. This model represents a tenfold scale-up from previous research and demonstrates that large-scale model training is no longer exclusive to large corporations. It shows that distributed, community-driven approaches can achieve similar outcomes, paving the way for even larger model sizes and the eventual goal of open-sourcing AI general (AGI).
Along with releasing the INTELLECT-1 model, intermediate checkpoints, post-trained models, and a detailed technical report, the team also provides a chat interface, pre-training datasets, and post-training datasets collaboratively developed with Arcee AI.
The collaborative training of INTELLECT-1 involved the innovative use of decentralized resources, spanning five countries and three continents.
The model was trained over 1 trillion tokens using up to 112 H100 GPUs simultaneously. Despite the geographically distributed nature of the training, the system achieved 83% overall compute utilization across continents and 96% compute utilization within the United States, with a median sync time of 103 seconds within the U.S. and 469 seconds globally, demonstrating minimal overhead compared to centralized training approaches.
These results highlight the feasibility of decentralized training for large-scale foundation models, even in the face of bandwidth constraints and node volatility.
The key technical innovations that enabled this achievement were developed through PRIME, the distributed training framework.
PRIME features innovations such as ElasticDeviceMesh, which supports dynamic global process groups for fault-tolerant communication over the internet and local process groups for communication within a node. Additionally, PRIME supports live checkpoint recovery, custom kernels, and a hybrid DiLoCo-FSDP2 implementation. Together, these innovations enabled a 400x reduction in communication bandwidth compared to traditional data-parallel training approaches, while maintaining performance at the 10B scale.
INTELLECT-1 Specifications
INTELLECT-1 is built upon the large language model meta AI version 3 (Llama-3) architecture, consisting of 42 layers with 4,096 hidden dimensions, 32 attention heads, and an 8,192 sequence length. The model uses a vocab size of 128,256 and was trained on a curated dataset containing 1 trillion tokens.
The dataset mix includes 55% FineWeb-Edu, 20% stack v2, 10% FineWeb, 10% deep contrastive learning model (DCLM)-baseline, and 5% OpenWebMath.
The training took place over 42 days and utilized various techniques, including a word sense disambiguation (WSD) learning rate scheduler, a 7.5e-5 inner learning rate, auxiliary max-z-loss for stability, and Nesterov momentum as the outer optimizer. The dynamic onboarding and offboarding of compute resources allowed the system to scale up to 14 nodes during training.
The system demonstrated remarkable computing efficiency throughout the training process across various geographical configurations. For transatlantic training, compute utilization reached 85.6%, with a median sync time of 382 seconds. In the globally distributed setting, the system maintained 83% compute utilization, with a median sync time of 469 seconds, showcasing the efficiency of PRIME's decentralized architecture in diverse training environments.
Post-Training Enhancements
After completing the pre-training phase, INTELLECT-1 underwent a series of post-training enhancements in collaboration with Arcee AI to improve its task-specific performance.
This post-training phase involved three essential techniques: extensive supervised fine-tuning (SFT) with 16 runs, decision policy optimization (DPO) with eight runs, and strategic model merging using MergeKit. These techniques were designed to fine-tune the model's capabilities for specific tasks, ensuring that INTELLECT-1 performed optimally across various applications. This collaborative effort showcases the power of community-driven AI advancements.
Conclusion
In conclusion, the successful release and training of INTELLECT-1 marks a significant milestone in decentralized AI development. By leveraging the PRIME framework, this work demonstrates the potential for distributed, community-driven model training on a global scale, opening up new possibilities for the future of AI research and development.
Looking ahead, the team aims to scale up this approach to even larger models, implement new economic incentives to foster community participation and continue optimizing the distributed architecture to achieve breakthroughs in AI capabilities. The open sourcing of INTELLECT-1 serves as an invitation for the global AI community to collaborate, pushing the boundaries of decentralized training together.