New research unveils a breakthrough in spotting when AI-generated images mimic real artists too closely, giving developers tools to avoid copyright traps and push ethical boundaries.
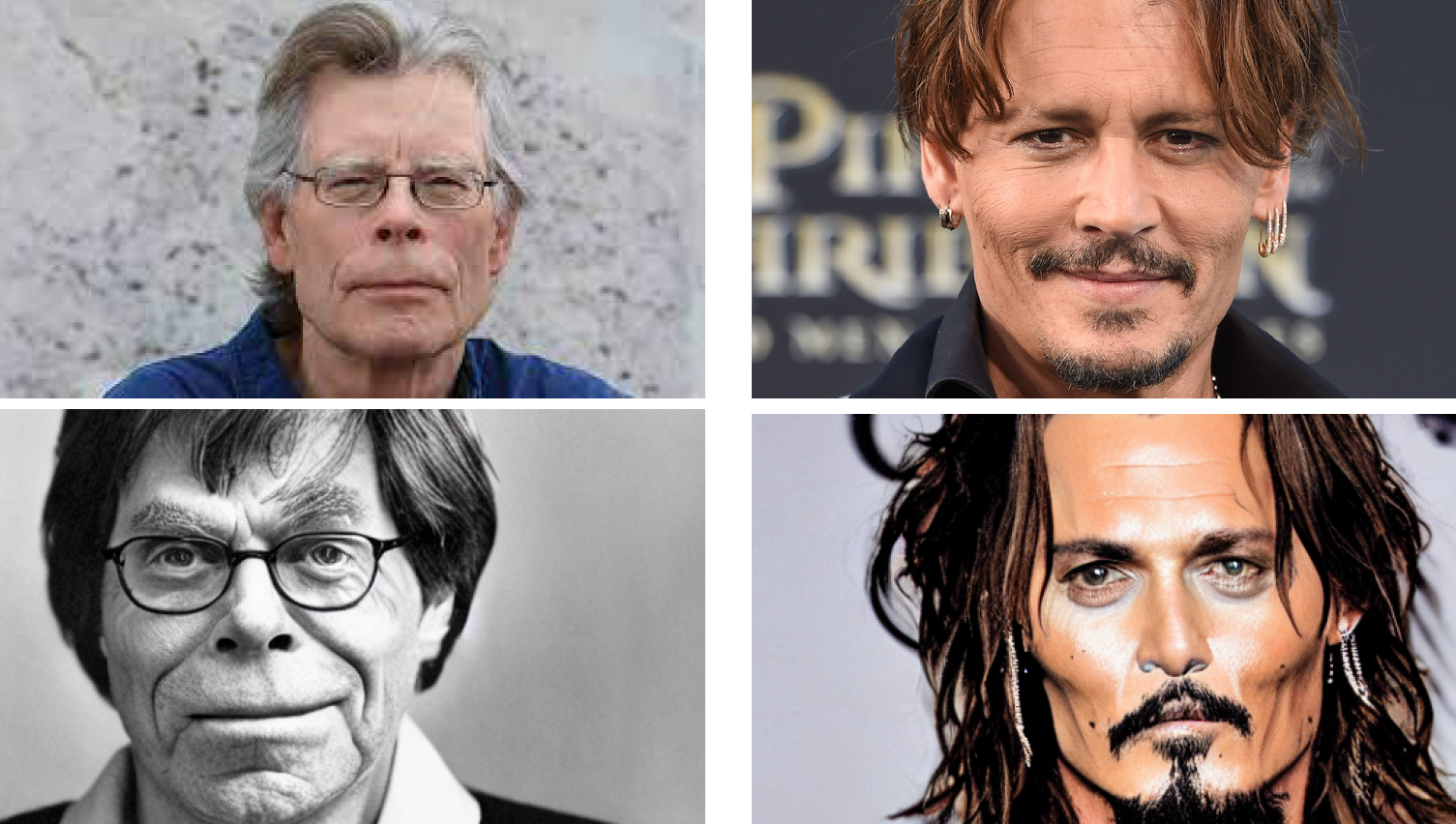
Research: How Many Van Goghs Does It Take to Van Gogh? Finding the Imitation Threshold

*Important notice: arXiv publishes preliminary scientific reports that are not peer-reviewed and, therefore, should not be regarded as definitive, used to guide development decisions, or treated as established information in the field of artificial intelligence research.
A research paper recently posted to the arXiv preprint server examined the implications of training text-to-image models on datasets containing copyrighted and private content. It focused on a critical issue in artificial intelligence (AI), specifically, the phenomenon of imitation, where models may unintentionally replicate specific art styles or images closely resembling/matching their training data.
The researchers introduced a novel technique called "Measuring Imitation Threshold Through Instance Count and Comparison (MIMETIC2)" to determine the minimum number of instances of a visual concept needed for models to imitate it effectively. They defined this minimum level as the "imitation threshold," a key metric for understanding how well a model can replicate artistic styles or specific images.
"MIMETIC2 leverages a change detection algorithm, known as PELT, which pinpoints the point at which a model’s ability to imitate undergoes a notable shift, establishing the imitation threshold."
Advancement in Image Generative Technology
In recent years, multi-modal vision-language models have advanced significantly due to large datasets like LAION, which contain image-text pairs collected from the internet. These datasets enable models to learn and generate images from text descriptions.
Text-to-image models, particularly those using diffusion techniques, are now state-of-the-art for image generation. These models learn to create images by recognizing data patterns based on text inputs. However, concerns have emerged regarding including private, copyrighted, and licensed material in training data, leading to copyright and privacy issues.
These concerns have raised legal and ethical challenges, as artists and individuals worry about unauthorized use of their work or likeness. Therefore, understanding the extent of imitation in these models and establishing thresholds for when they can accurately reproduce a concept is crucial for complying with copyright and privacy laws.
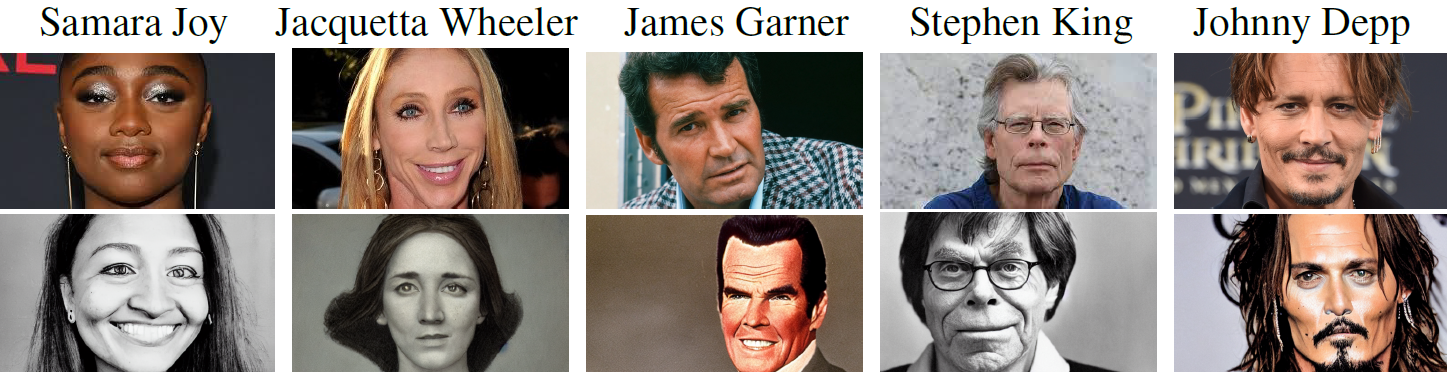
Examples of real celebrity images (top) and generated images from a text-to-image model (bottom) with increasing image counts from left to right (3, 273, 3K, 10K, and 90K, respectively). The prompt is “a photorealistic close-up image of {name}”.
Investigating the Imitation Threshold Using MIMETIC2
The paper introduced a novel problem called "Finding the Imitation Threshold (FIT)" to estimate the minimum number of examples needed for a text-to-image model to imitate visual concepts without retraining new models from scratch. The authors proposed the MIMETIC2 method, an innovative approach that estimates the imitation threshold using existing models and observational data.
The MIMETIC2 approach involves collecting concepts within specific domains, such as human faces and art styles, and generating images using pre-existing text-to-image models. The researchers compiled datasets with different concepts and recorded how often each concept appeared in the training data. They then generated images for each concept and calculated an imitation score by comparing these generated images to the original training images.
This imitation score was verified with human evaluations, ensuring that the results aligned with human perceptions of similarity. By sorting concepts based on frequency and using a change detection algorithm, they identified the point at which the model reliably imitates a concept, marking it as the imitation threshold.
The study used three versions of the Stable Diffusion (SD) model, including SD1.1, SD1.5, and SD2.1, trained on the LAION2B-en and LAION5B datasets. The experiments focused on two domains: human faces and art styles. The paper emphasizes that the larger LAION5B dataset tends to produce higher imitation thresholds, suggesting that dataset size correlates with imitation capacity. The framework provides valuable insights into how the frequency of training data affects a model’s imitation capabilities.
An overview of FIT, where we seek the imitation threshold – the point at which a model was exposed to enough instances of a concept that it can reliably imitate it. The figure shows four concepts (e.g., Van Gogh’s art style) that have different counts in the training data (e.g., 213K for Van Gogh). As the image count of a concept increases, the ability of the text-to-image model to imitate it increases (e.g. Piet Mondrian and Van Gogh). We propose an efficient approach, MIMETIC2, that estimates the imitation threshold without training models from scratch.
Key Outcomes and Insights
The results showed that imitation thresholds ranged from 200 to 600 images, depending on the domain and model. For example, SD1.1 required 364 images for celebrities and 234 for politicians. In the realm of art styles, the thresholds were lower, with classical artists at 112 images and modern artists at 198. SD2.1, trained on the larger LAION5B dataset, demonstrated higher imitation thresholds across these categories.
Additionally, the authors found that the models were less likely to imitate certain concepts with fewer instances in the training data. This finding has significant implications for copyright and privacy, indicating that concepts with limited representation are less likely to be imitated. Furthermore, the study emphasized the importance of understanding the relationship between training data frequency and model behavior, providing a framework for evaluating copyright concerns.
Applications
Identifying imitation thresholds has important implications for generative models' ethical and legal development. Developers can use these thresholds to manage training datasets better and avoid excessive similarity to copyrighted or personal images, reducing the risk of violations. This approach offers a standard for addressing intellectual property and privacy issues and provides a way to assess model compliance.
Setting imitation threshold guidelines could encourage the development of models that use a broader range of data rather than repeated instances of the same concept. This could foster AI creativity while minimizing legal and ethical concerns. Additionally, this research can support legal frameworks for using copyrighted material in training datasets, contributing to the creation of ethical standards in AI and machine learning.
MIMETIC2's approach assumes that each image in a dataset contributes equally to concept learning and relies on statistical change detection to infer thresholds accurately. These assumptions, while effective in this study, indicate areas for further refinement.
Generated images of Samuel L. Jackson that show the model has captured a specific characteristic of his face (middle-aged, bald, with no or little beard).
Conclusion and Future Directions
In summary, this study provided valuable insights into the imitation behavior of text-to-image models, highlighting how the frequency of concepts in training datasets affects the models' ability to replicate them. By introducing the imitation threshold and MIMETIC2, it offers a practical framework for assessing potential copyright concerns and guiding ethical practices in generative model development.
Future work should focus on refining methods to assess imitation thresholds for underrepresented artistic styles and cultural artifacts. Further investigation into model architectures and training techniques could reveal additional factors influencing imitation capabilities. Collaboration between technologists, legal experts, and artists will be crucial for creating frameworks that protect intellectual property while fostering innovation in AI.

*Important notice: arXiv publishes preliminary scientific reports that are not peer-reviewed and, therefore, should not be regarded as definitive, used to guide development decisions, or treated as established information in the field of artificial intelligence research.
Journal reference:
- Preliminary scientific report.
Verma, S., & et al. How Many Van Goghs Does It Take to Van Gogh? Finding the Imitation Threshold. arXiv, 2024, 2410, 15002. DOI: 10.48550/arXiv.2410.15002, https://arxiv.org/abs/2410.15002