Apollo’s innovative approach to audio restoration significantly boosts audio quality by preserving low-frequency components and accurately reconstructing mid-to-high frequencies, setting a new benchmark for high-quality, real-time audio restoration.
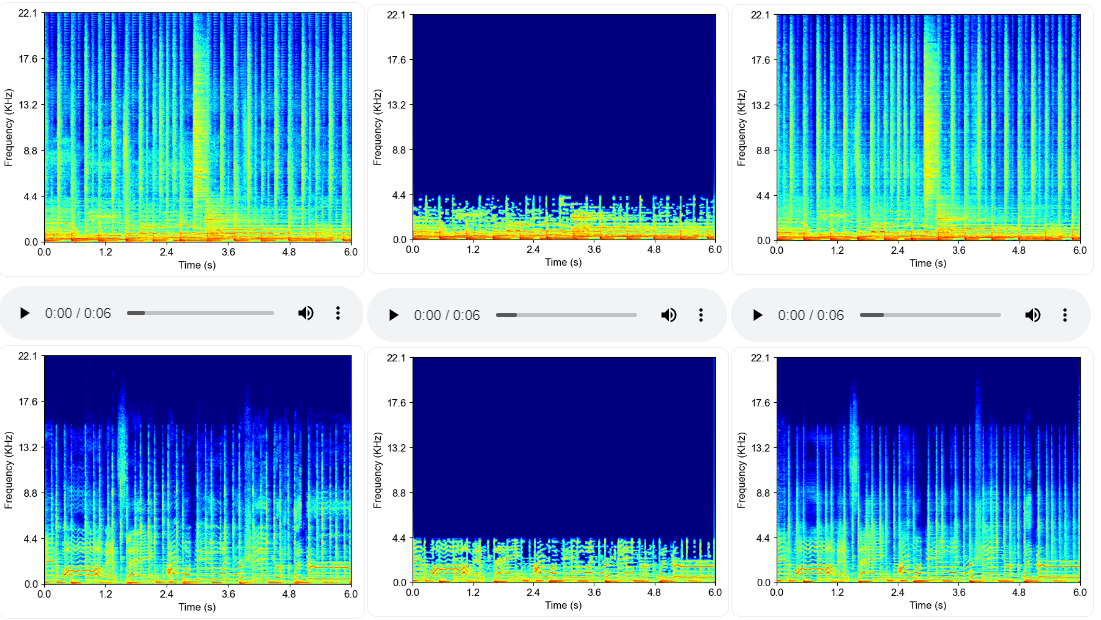
Study: Apollo: Band-sequence Modeling for High-Quality Audio Restoration

*Important notice: arXiv publishes preliminary scientific reports that are not peer-reviewed and, therefore, should not be regarded as definitive, used to guide development decisions, or treated as established information in the field of artificial intelligence research.
In an article submitted to the arXiv preprint* server, researchers introduced Apollo, a novel generative model for high-sample-rate audio restoration. Apollo employs an explicit frequency band split module to address challenges in accurately preserving low-frequency information while reconstructing high-quality mid- and high-frequency content.
When evaluated on the integrated music source separation dataset 18 - high quality (MUSDB18-HQ) and MoisesDB datasets, Apollo not only outperformed existing super-resolution generative adversarial network (SR-GAN) models, but it also excelled in complex music scenarios, significantly enhancing restoration quality with greater computational efficiency and a more compact model size.
Background
Past work in audio restoration has focused on rejuvenating vintage music and improving speech communication by repairing degraded audio. Techniques like bandwidth extension aim to reconstruct high-frequency information but often introduce artifacts.
Recent advances use GANs for more balanced audio quality and restoration, balancing perceptual quality with distortion. Building on these advancements, the Apollo model incorporates frequency band split and sequence modeling modules to not only handle high-sample-rate audio restoration but also effectively address complex acoustic characteristics, ensuring low-frequency preservation and the reconstruction of clear mid- and high-frequency details.
Apollo Restoration Method
The Apollo model employs a multi-stage approach to high-sample-rate audio restoration by integrating several key modules. It begins with a frequency band split module, which divides the audio spectrogram into sub-band spectrograms with predefined bandwidths. This step allows the model to analyze and process different frequency ranges separately while preserving global frequency dependencies.
Following the split, the Apollo model uses a frequency band sequence modeling module to capture relationships between sub-band frequency bands and their sequences. This module utilizes the Roformer and temporal convolutional networks (TCNs) to efficiently model frequency and temporal features, enabling more accurate audio restoration.
The final stage involves a frequency band reconstruction module that maps the extracted features through stacked nonlinear layers to produce the restored sub-band spectrograms. This process ensures that low-frequency components are preserved while the model reconstructs high-quality mid- and high-frequency details across multiple spectral resolutions.
Additionally, Apollo’s architecture supports streaming processing, enabling efficient real-time audio restoration. By incorporating both causal convolution and causal Roformer, the model maintains computational efficiency and adaptability, making it suitable for practical applications that require immediate audio enhancement.
Apollo Evaluation Results
The Apollo model was trained and tested using the combined MUSDB18-HQ and MoisesDB datasets to evaluate its performance across a diverse range of music genres. This integration allowed for a more comprehensive evaluation of Apollo's restoration capabilities.
During data preprocessing, a source activity detector (SAD) was employed to remove silent regions from the tracks, focusing the training on significant portions. Real-time data augmentation was applied by randomly mixing tracks from different songs, scaling energy levels within a range of [-10, 10] dB, and simulating dynamic bitrate scenarios using MP3 codecs with bitrates ranging from 24,000 to 128,000.
Careful tuning of the hyperparameters for the Apollo model was crucial to its optimized performance. The short-time Fourier transform (STFT) window length was set to 20 ms with a hop size of 10 ms using a Hanning window. Frequency band segmentation was configured with a bandwidth of 160 Hz and a feature dimension of 256.
The band sequence modeling module was stacked six times, and a multi-scale STFT window setup was used in the discriminator network. The generator and discriminator utilized the AdamW optimizer with specific learning rates and weight decay, and an early stopping mechanism was implemented to prevent overfitting. Training was conducted on a high-performance setup consisting of eight Nvidia RTX 4090 GPUs.
Evaluation metrics included the scale-invariant signal-to-noise ratio (SI-SNR), signal-to-distortion ratio (SDR), and virtual speech quality objective listener (VISQOL) scores to assess audio quality. The Real-Time Factor (RTF) was measured to evaluate processing efficiency, calculating the time per second of audio processed on both the central processing unit (CPU) and the graphics processing unit (GPU). The team assessed model size by reporting the number of parameters using PyTorch-OpCounter.
Apollo's restoration performance was compared with SR-GAN across different bitrates and music genres. Results indicated that Apollo consistently outperformed SR-GAN, especially in handling frequency band voids and reduced signal bandwidth, as reflected in higher SI-SNR and SDR scores. Apollo also improved audio restoration quality, as indicated by virtual speech quality objective listener (VISQOL) scores.
Further analysis revealed Apollo's superiority in various music genres, including vocals, single instruments, mixed instruments, and combinations of instruments with vocals. Apollo's unique alternating band and sequence modeling architecture provided an advantage in complex scenarios with mixed instruments and vocals. Compared to SR-GAN, Apollo delivered not only higher user ratings but also comparable inference speed with a significantly more compact model size, making it particularly effective for real-time communications and live audio restoration.
Conclusion
To sum up, Apollo represents a breakthrough in compressed audio restoration. It significantly enhances audio quality through its band split, sequence modeling, and reconstruction modules. Empirical evaluations on the MUSDB18-HQ and MoisesDB datasets confirmed Apollo’s exceptional performance across diverse genres and compression levels.
The model not only substantially improved music restoration but also maintained a smaller size and achieved high computational efficiency. Experimental results demonstrated that Apollo’s band split and band-sequence modeling effectively captured and restored intricate audio information lost during compression, addressing the most challenging acoustic characteristics.

*Important notice: arXiv publishes preliminary scientific reports that are not peer-reviewed and, therefore, should not be regarded as definitive, used to guide development decisions, or treated as established information in the field of artificial intelligence research.
Source:
- “Apollo: Band-Sequence Modeling for High-Quality Music Restoration in Compressed Audio.” Cslikai.cn, 2024, cslikai.cn/Apollo/.
Journal reference:
- Preliminary scientific report.
Li, K., & Luo, Y. (2024). Apollo: Band-sequence Modeling for High-Quality Audio Restoration. ArXiv. DOI:10.48550/arXiv.2409.08514, https://arxiv.org/abs/2409.08514v1