Explore how AI is reshaping fashion by generating personalized, trend-setting outfits that set new standards in the industry.
Study: Prompt2Fashion: An automatically generated fashion dataset. Image Credit: Shutterstock AI Generator

*Important notice: arXiv publishes preliminary scientific reports that are not peer-reviewed and, therefore, should not be regarded as definitive, used to guide development decisions, or treated as established information in the field of artificial intelligence research.
In an article recently posted on the arXiv* preprint server, researchers explored the intersection of artificial intelligence (AI) and fashion by developing a comprehensive fashion image dataset called "Prompt2Fashion" using generative models. Their goal was to bridge the gap between personalized fashion needs and AI-driven design, providing a scalable solution for producing high-quality, diverse fashion images suited to various occasions, styles, and body types.
Background
Integrating AI in the fashion industry transforms creativity, personalization, and efficiency. AI is used to design garments, predict trends, and improve various aspects of fashion design and marketing. However, there are challenges in assessing AI-generated content.
Traditional metrics like Fréchet Inception Distance and Inception Score measure the quality and diversity of the image but do not capture fashion-specific features or attributes, such as trend relevance, style consistency, and aesthetic appeal. This highlights the need for domain expertise to evaluate AI-generated fashion content to meet consumer expectations and industry standards.
About the Research
In this paper, the authors aimed to address the lack of comprehensive datasets for personalized fashion by using Large Language Models (LLMs) and Diffusion Models to generate fashion images. They developed a novel dataset of fashion outfits that are entirely AI-generated, eliminating the need for existing annotated pictures. This method allows for creating a wide range of diverse images that meet different standards and personalization needs, which would be expensive and time-consuming using traditional methods.
The dataset includes various characteristics, such as gender (male, female), body type, occasions, styles, and their combinations. The researchers used LLMs like Falcon-7B and Mistral-7B to generate descriptions, which were input into a Stable Diffusion model to create the final images.
The input to the model consisted of variable triplets like "style, occasion, gender" and "style, occasion, type," enabling the model to adapt to different body types and genders. The final dataset contains 2,000 samples, each including the original triplet, LLM output, and the diffusion model output image.
Research Findings
The outcomes showed that the generated images and descriptions were both relevant and aesthetically pleasing. Non-expert human evaluators provided detailed feedback on the quality and relevance of the generated outfits. The style of the outfits received an average rating of 4.1 out of 5, indicating that most participants found that the styles matched/aligned with the intended designs.
The alignment of outfits with the wearer's type received the highest average rating of 4.4, reflecting strong agreement on this match. Aesthetic appeal, creativity, and coherence were rated from moderate to very creative, with nearly all participants agreeing that the garments and accessories complemented each other well.
However, the study also emphasized the importance of expert evaluation for AI-generated fashion content. While non-expert feedback confirmed the appeal of the generated images, expert evaluations provided a deeper and more consistent perspective. Experts assessed trend relevance, style consistency, cultural sensitivity, and technical details like fabric representation and garment construction. Their input ensured that the generated designs met high artistic standards and were suitable for real-world applications.
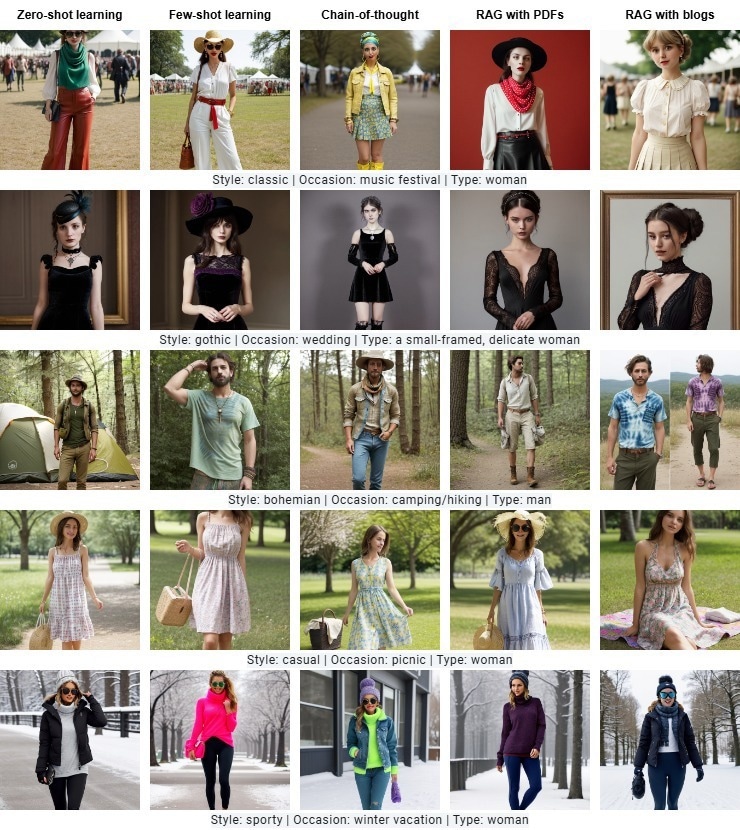
Applications
The developed dataset has several potential applications across different fields. For example, engineers testing models can use the diverse representations of individuals and scenarios to ensure adaptability and robustness in the image generation models. Designers can leverage the dataset to display various ethnicities, fashion styles, and body types in different contexts, helping create more inclusive product designs and marketing materials.
Advertising and marketing professionals and agencies can utilize the detailed contexts of the images to improve narrative elements and storytelling to develop more engaging ads. Academic researchers analyzing consumer behavior and fashion trends can use the generated dataset to explore how various factors influence preferences and perceptions.
Conclusion
The LLM and Diffusion Model-based approach proved effective and robust for accurately generating a novel and comprehensive fashion dataset. The images and descriptions were relevant and visually appealing, as confirmed by non-expert feedback. However, the authors emphasized the need for expert evaluation to ensure high fashion quality and marketability standards.
Future work should incorporate expert ratings to refine the dataset further and improve its usefulness. Additionally, integrating more advanced generative models and expanding the dataset to include more diverse fashion elements could increase its applicability.
Collaborating with fashion industry professionals for real-world testing and feedback would also be valuable. Overall, this dataset represents a significant advancement in AI-driven fashion design, providing a valuable resource for future research and industry applications.

*Important notice: arXiv publishes preliminary scientific reports that are not peer-reviewed and, therefore, should not be regarded as definitive, used to guide development decisions, or treated as established information in the field of artificial intelligence research.
Journal reference:
- Preliminary scientific report.
Argyro, G., & et, al. Prompt2Fashion: An automatically generated fashion dataset. arXiv, 2024, 2409, 06442v1. DOI: 10.48550/arXiv.2409.06442, https://arxiv.org/abs/2409.06442v1